Wikis
Info-nuggets to help anyone understand various concepts of MLOps, their significance, and how they are managed throughout the ML lifecycle.
Stay up to date with all updates
Privacy Evaluation
Assessing how well a model or dataset protects sensitive information
Privacy Evaluation in the context of data analysis and machine learning involves assessing how well a model or dataset protects sensitive information. This can be approached through various frameworks that focus on different aspects of privacy, such as univariate and multivariate evaluations, linkability, and inference attacks. Here’s an overview of each of these concepts:
1. Univariate Privacy Evaluation
Univariate privacy evaluation focuses on assessing the privacy risks associated with individual attributes or features within a dataset. The main goal is to determine how well sensitive information is protected when considering one variable at a time.
Key Concepts:
- Data Sensitivity: Identifies which features are sensitive and how their disclosure could impact individuals.
- Statistical Disclosure Control: Techniques like noise addition, data masking, or generalization to protect sensitive features.
- Utility vs. Privacy Trade-off: Balancing the accuracy of the data analysis with the need to protect individual privacy.
2. Multivariate Privacy Evaluation
Multivariate privacy evaluation examines privacy risks associated with the relationships between multiple attributes simultaneously. This approach is crucial because privacy threats can arise not just from individual features but from their interactions.
Key Concepts:
- Joint Distribution: Analyzing how the combination of multiple attributes can increase the risk of re-identification.
- Correlation and Dependencies: Understanding how closely related features can be used together to infer sensitive information about individuals.
- K-anonymity and L-diversity: Techniques that aim to ensure that data records are indistinguishable from at least K other records in the dataset or that sensitive attributes have diverse values within groups.
3. Linkability
Linkability refers to the ability to link records or data points to the same individual across different datasets or over time. This poses significant privacy risks, particularly in longitudinal studies or when integrating data from multiple sources.
Key Concepts:
- Re-identification Risk: The probability that individuals can be re-identified by linking data points across different datasets or by connecting data across time.
- Data Integration: Understanding how combining datasets (e.g., public records with private data) increases the risk of linking sensitive information back to individuals.
- Differential Privacy: A mathematical framework that ensures that the output of a database query does not significantly change when a single record is added or removed, thereby reducing linkability.
4. Inference
Inference refers to the ability to draw conclusions or make predictions about sensitive information based on available data, which could lead to unauthorized access to private information.
Key Concepts:
- Attribute Inference: The risk of inferring sensitive attributes of individuals based on other non-sensitive attributes available in the dataset.
- Membership Inference: Determining whether a particular individual was part of the training dataset used for a model, which can reveal sensitive information.
- Attack Models: Understanding various methods (e.g., background knowledge, adversarial models) that can be used to make inferences about sensitive data.
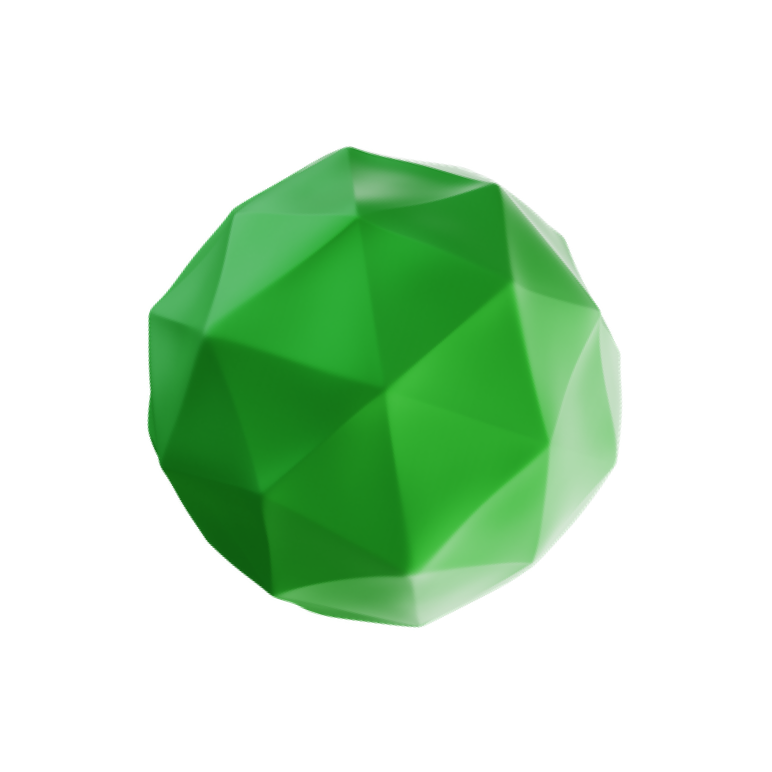
Is Explainability critical for your AI solutions?
Schedule a demo with our team to understand how AryaXAI can make your mission-critical 'AI' acceptable and aligned with all your stakeholders.