Wikis
Info-nuggets to help anyone understand various concepts of MLOps, their significance, and how they are managed throughout the ML lifecycle.
Model Drift
Model drift transpires when the ML models predictive ability "drifts" from the performance over the training period.
Model drift transpires when the ML models predictive ability "drifts" from the performance over the training period. It is a deterioration of the model’s predictive ability, where the model makes less accurate predictions from incoming values when compared to its original prediction. Monitoring for drift is crucial in machine learning observability, enabling teams to quickly diagnose production issues that adversely affect your model’s performance.
Model drift can happen for a variety of issues, including the following:
- Data drift: Change in statistical properties of independent variables. There exists a substantial gap between the time of the gathering of the data and when the model is applied to predict real data.
- Concept drift: Change in Actuals - the correlation between input and target variables changes
- Upstream data changes: When the relationship between input and target variables changes
Techniques to measure data drift:
- Kullback-Leibler (KL) divergence
- Population Stability Index (PSI)
- Jensen-Shannon (JS) Divergence
- Chi-square test
- Z-Test
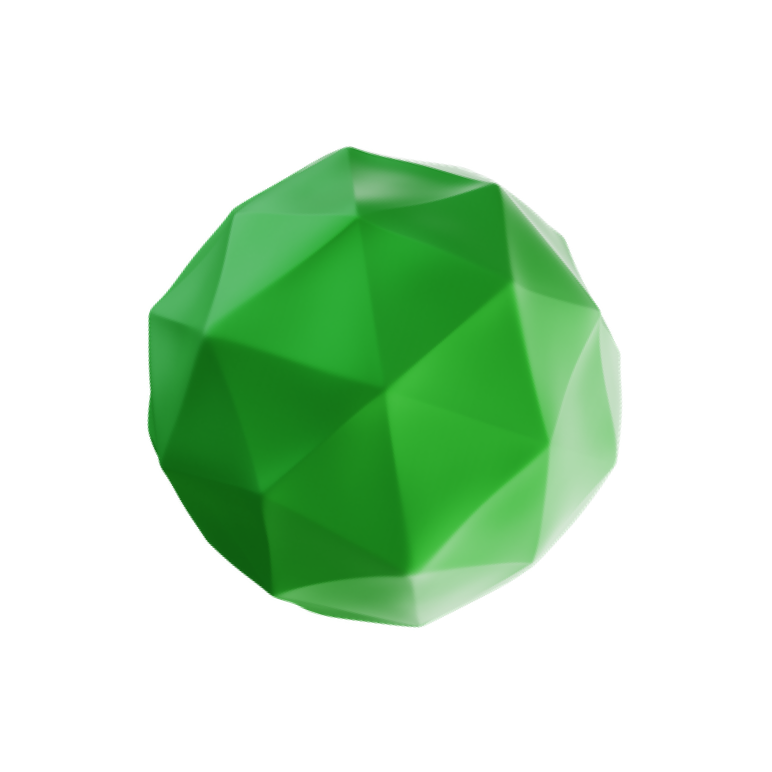
Is Explainability critical for your AI solutions?
Schedule a demo with our team to understand how AryaXAI can make your mission-critical 'AI' acceptable and aligned with all your stakeholders.