India’s Strategic Leap Toward Responsible AI: The IndiaAI Safety Institute
8 minutes
April 28, 2025
%20-%20AryaXAI%20blog.png)
As artificial intelligence continues to rapidly evolve, the conversation around its responsible development and deployment has become increasingly urgent. The institute aims to shape the future of AI development and deployment in India, ensuring that technological advancements benefit society as a whole. Global technology companies like Apple and Google are also advancing responsible AI, and India’s initiative positions it alongside these leaders in the tech industry. This mirrors the historical influence of trading companies such as the East India Company, which played a pivotal role in shaping economic and technological development in India. In January 2025, India took a significant step toward leading this global dialogue by announcing plans to establish the IndiaAI Safety Institute (AISI). Spearheaded by the Ministry of Electronics and Information Technology (MeitY) under the broader IndiaAI Mission, this initiative marks a landmark effort to ensure that AI technologies developed and deployed within the country are safe, ethical, and aligned with national priorities.
The institute will serve as a cornerstone of the Safe and Trusted AI pillar within the IndiaAI Mission and seeks to build an ecosystem where innovation coexists with accountability, transparency, and public trust. Integrating responsible AI principles into business operations will be a key focus, fostering trust and compliance for Indian enterprises. However, it is important to consider the economic and infrastructural cost of implementing responsible AI practices and establishing such an institute. Its mission is crucial for ensuring safe, ethical, and trustworthy AI in India.
The Vision and Framework: Building a Contextual AI Safety Ecosystem
The IndiaAI Safety Institute has been conceptualized to act as a central authority on AI safety, risk mitigation, and responsible AI deployment. It is structured around a hub-and-spoke model, where a central institution collaborates with a network of academic institutions, research organizations, startups, industry players, and other organizations involved in India's socio-economic development.
This model enables both centralized coordination and decentralized execution. For example, while the central institute may define the safety standards and research priorities, which are centrally controlled to ensure consistency and governance, specialized research labs at IITs or private AI firms may execute projects in their areas of expertise. The hub-and-spoke model supports continuous processes of research, development, and oversight, ensuring that human involvement and monitoring are maintained throughout the AI lifecycle. This allows for a highly adaptive system that evolves with the technology landscape.
A critical strength of this initiative is its focus on indigenous R&D using Indian datasets. AI systems built and trained exclusively on Western datasets often fail to perform accurately in Indian contexts due to vast linguistic, cultural, and socio-economic differences. For instance, a facial recognition algorithm trained on Western datasets may exhibit significantly lower accuracy when identifying faces from diverse Indian ethnic groups. By emphasizing research tailored to Indian realities, the institute aims to close these gaps and build inclusive AI systems. Integrating responsible AI practice, such as explainability and safety techniques, into the development of contextually relevant models is essential for building trust, ensuring transparency, and meeting ethical standards. Explainability is particularly important as it enables human users to comprehend how AI decisions are made, which helps build trust and allows for better evaluation of AI strengths and limitations.
First Round of Responsible AI Projects: From Theory to Application
To kickstart the Safe and Trusted pillar, IndiaAI launched its first Expression of Interest (EoI) in late 2024, which saw an overwhelming response from the academic and industrial research community. From over 2,000 applications, eight projects were selected based on their relevance, feasibility, and alignment with national AI safety priorities. These projects reflect a diverse set of problem statements, ranging from technical challenges to ethical frameworks. Collectively, they advance responsible AI practices by integrating comprehensive technical and ethical approaches throughout the AI lifecycle. The expected impact of these projects includes setting new benchmarks for responsible AI development in India and contributing to anticipated improvements in AI safety and governance.
1. Machine Unlearning – IIT Jodhpur
As data privacy norms tighten globally, the concept of machine unlearning has gained importance. It refers to the ability of a model to forget specific data points, ensuring that users can exercise their “right to be forgotten.” These techniques give users greater control over their personal data in AI systems, allowing them to decide what information is retained or removed to better protect sensitive information and comply with regulations. For example, if an individual withdraws consent for their medical data to be used in training an AI diagnostic tool, machine unlearning would ensure the model no longer retains or uses that data.
2. Synthetic Data Generation – IIT Roorkee
Given the scarcity of high-quality, annotated datasets in regional languages and domains like agriculture or informal labor, generating synthetic datasets has become essential. IIT Roorkee is working on methods to produce synthetic data generation methods that can help train AI systems in low-data environments without compromising on privacy. The output created by these synthetic data generation methods not only enhances model robustness but also supports the development of explainable AI by making it easier to analyze and interpret how models learn from and respond to diverse data scenarios.
3. Bias Mitigation in AI Models – NIT Raipur
AI models often inadvertently perpetuate societal biases. A recruitment tool trained on historical hiring data might systematically disadvantage women or marginalized communities. NIT Raipur’s project explores algorithmic techniques to detect and mitigate such biases before they become embedded in AI systems. Monitoring model performance is essential for detecting and addressing bias, as it allows for the evaluation of accuracy and fairness throughout the AI lifecycle.
4. Privacy-Enhancing Technologies – DIAT Pune and Mindgraph
This collaboration focuses on cryptographic methods like homomorphic encryption and federated learning, which allow AI models to be trained without direct access to sensitive data. Adhering to established privacy principles is essential in guiding the design and implementation of these privacy-enhancing technologies, ensuring compliance with regulatory frameworks and robust data protection. This is particularly relevant in sectors such as healthcare, where patient confidentiality is paramount.
5. Explainable AI for Public Services – IIT Delhi, IIT Dharwad, IIIT Delhi
As government services increasingly rely on AI (e.g., predictive policing or welfare benefit distribution), ensuring that decisions made by these systems are understandable and auditable is essential. These institutions are developing explainability frameworks to make AI decisions transparent to both administrators and citizens. Key features of these frameworks include transparency, traceability, and a focus on human factors that contribute to trustworthy and interpretable AI systems. Explanations provided by these frameworks can take various forms, such as visual, textual, or interactive formats, depending on the audience and purpose. These frameworks are specifically designed to help human users, including non-technical stakeholders, understand and trust AI decisions.
6. Ethical AI Frameworks for Governance – IIIT Delhi
This project aims to translate abstract ethical principles into actionable policies for public sector AI use. To address ethical concerns, the project incorporates monitoring, auditing, and accountability mechanisms to ensure responsible AI deployment. For example, guidelines on consent management, data provenance, and algorithmic fairness for government departments using AI in education or transportation.
7. Algorithm Auditing Tools – Civic Data Labs
Independent auditing is necessary to verify whether deployed AI systems comply with ethical norms and legal frameworks. Civic Data Labs is developing open-source tools that allow such audits to be conducted efficiently, making AI systems more accountable to the public. These open-source auditing tools contribute to increased transparency and accountability in AI deployments, helping users better understand how AI models function and ensuring responsible use.
8. AI Risk Testing Frameworks – Amrita Vishwa Vidyapeetham
Similar to stress testing in the financial sector, this project aims to develop a comprehensive framework for testing AI systems under high-risk scenarios, such as adversarial attacks or deployment in critical infrastructure. It is essential to identify and mitigate risks associated with deploying AI in critical infrastructure to ensure safety, security, and regulatory compliance.
Second Expression of Interest: Scaling the Mission
Following the momentum of the first EoI, IndiaAI launched the second round of EoI in early 2025, broadening the scope and inviting participation from a wider pool of Indian academic institutions, startups, and R&D organizations. This second EoI aims to accelerate the adoption of responsible AI across sectors, ensuring that AI and machine learning technologies are integrated and accepted widely. A diverse range of organizations—including government bodies, international agencies, and non-governmental entities—are participating in this initiative. These organizations contribute to the development and implementation of responsible AI protocols, supporting ethical and effective AI deployment. Reports generated by participating organizations help communicate complex findings and model explanations to stakeholders, enhancing transparency and understanding. Additionally, stories of successful AI deployments are shared to inspire further innovation and adoption within the community.
The themes in this round are deeply strategic and forward-looking:
- Deepfake Detection: In light of the increasing misuse of AI-generated videos in misinformation campaigns, this initiative seeks technical tools for early detection and provenance verification.
- Stress Testing Tools: Ensuring the robustness of AI systems, especially those used in mission-critical applications such as disaster response or national security.
- AI Risk Assessment & Management: Developing comprehensive risk taxonomies and mitigation strategies applicable across industries.
- Watermarking and Labelling: Techniques to label AI-generated content to prevent confusion or misuse, particularly on social media.
- Responsible Use Protocols: Guidelines and compliance frameworks that organizations must adhere to when deploying AI technologies.
The deadline for submission was extended to February 28, 2025, signaling the government’s intent to make the process more inclusive and participatory. Project reviews and reporting cycles are typically scheduled in June and October to align with key milestones in the initiative. Regular reporting on project progress and outcomes is essential for transparency and stakeholder engagement, while ongoing reporting in technology news keeps the public informed about the latest developments and industry trends related to the initiative.
A Globally Aligned, Locally Rooted Approach
What sets the IndiaAI Safety Institute (AISI) apart from similar global initiatives is not only its ambition but its commitment to designing AI systems rooted in India’s cultural, linguistic, and socio-economic diversity. The AISI is also committed to developing trustworthy AI systems that meet global standards, with the ambition to position India among the world's leaders in responsible AI.
While countries across the world—from the EU’s AI Act to the U.S. Executive Order on AI—have made strides in establishing frameworks for responsible AI, India’s approach is uniquely positioned to reflect the realities of a complex, pluralistic society. The crucial role of local context in shaping effective responsible AI frameworks cannot be overstated, as it ensures solutions are tailored to India’s specific needs. Responsible AI frameworks help India realize the full potential of AI technologies by maximizing benefits and minimizing risks. The AISI aims to bridge the gap between global AI governance ideals and India’s grassroots-level deployment challenges, ensuring that safety, fairness, and trust are embedded in technologies meant for all layers of society.
Explainability and transparency in AI systems provide important insights for stakeholders and users, helping to build trust and support ethical and legal compliance.
1. Linguistic Diversity as an Inclusion Imperative
India is home to 22 constitutionally recognized languages, with thousands of dialects spoken across rural and urban landscapes. In contrast to markets where English or a single national language dominates, AI systems in India must function across multilingual environments—be it chatbots for agriculture advisory in Bundeli or healthcare applications offering instructions in Tamil.
This presents a multi-layered challenge for Natural Language Processing (NLP):
- Language equity: Prioritizing major Indian languages without marginalizing smaller dialects.
- Code-mixed language handling: Building models that understand text mixing Hindi and English (commonly used in messaging apps).
- Data collection and annotation: Creating high-quality, labeled datasets in underrepresented languages is often resource-intensive and commercially unappealing for global firms.
Grappling with these multilingual challenges provides valuable insight into the complexities of responsible AI development, helping stakeholders better understand and address linguistic diversity and inclusion.
The IndiaAI Safety Institute can play a critical role in developing foundational language models fine-tuned on Indic datasets, while also guiding ethical practices in regional language data sourcing.
2. Digital Literacy and Interface Design
The World Bank reports that nearly 40% of India’s population remains offline, and a significant portion of digital users have only basic literacy. This makes explainability and accessibility not just ideal traits—but absolute requirements.
For example:
- Voice-based navigation: AI platforms serving farmers, frontline health workers, or elderly users must go beyond text-heavy interfaces.
- Visual explanations: AI predictions—say, in weather forecasting or credit scoring—need to be backed with intuitive, image-based cues that are easy to grasp.
- Low-bandwidth optimization: AI apps must be lightweight, capable of running on entry-level smartphones without constant connectivity.
To address these challenges, XAI techniques—such as interactive interfaces, heat maps, and visualizations—are increasingly used to make AI systems more understandable and to build user trust.
In this context, the “explainable AI” principle becomes deeply local. It isn’t just about model interpretability for a data scientist—it’s about ensuring that a rural homemaker using an AI-enabled loan app understands why she was approved or denied credit.
The AISI is designed to support such socially rooted innovations, setting India-specific benchmarks for explainability, fairness, and usability.
3. Socio-economic Context as a Driver of Responsible AI
India’s diversity is not only linguistic—it encompasses caste, gender, income, geography, and education. Algorithms that work seamlessly in urban Bengaluru may fail in tribal Odisha or among underbanked communities in Bihar.
Globally, there’s growing concern about algorithmic bias. But in India, these concerns are heightened by structural inequalities that can be unintentionally reinforced if AI models are trained on non-representative or biased data. By their very nature, AI models are a form of statistical discrimination, which can influence issues like bias, fairness, and explainability. It is also crucial to monitor model accuracy to ensure that these systems deliver equitable and reliable outcomes for all groups.
For instance:
- A facial recognition system trained primarily on lighter-skinned individuals might underperform for darker-skinned populations, reinforcing exclusion in welfare schemes.
- An ed-tech recommendation engine that only suggests English-language courses could disadvantage students from vernacular backgrounds, despite equal potential.
The AISI’s mandate to work with contextualized Indian datasets ensures that responsible AI in India isn’t imported—it is co-designed with the communities it serves. These models are created in collaboration with local communities, increasing transparency and trust in the development process.
Conclusion: India as a Global Leader in Responsible AI
The establishment of the IndiaAI Safety Institute is a timely and strategic intervention. As countries worldwide debate regulations and ethical frameworks for AI, India has chosen to act by funding indigenous research, building institutional capacity, and nurturing a national ecosystem for AI safety and trust.
This is not just a policy announcement; it is the beginning of a paradigm where responsible innovation becomes the foundation of India's AI ambition. If successfully implemented, India may not only emerge as a leader in AI technology but also as a global benchmark in AI governance and ethics.
SHARE THIS
Discover More Articles
Explore a curated collection of in-depth articles covering the latest advancements, insights, and trends in AI, MLOps, governance, and more. Stay informed with expert analyses, thought leadership, and actionable knowledge to drive innovation in your field.
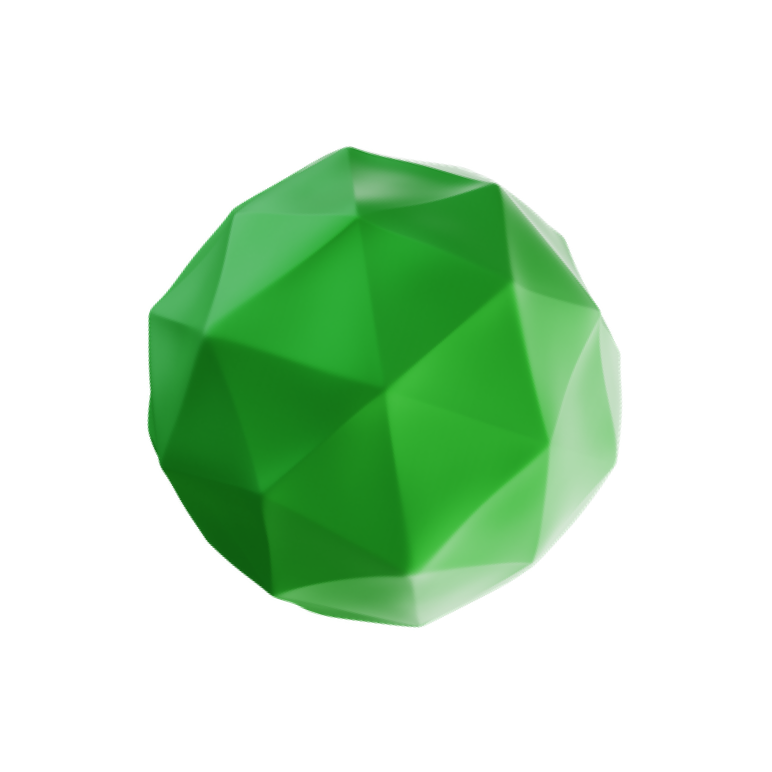
Is Explainability critical for your AI solutions?
Schedule a demo with our team to understand how AryaXAI can make your mission-critical 'AI' acceptable and aligned with all your stakeholders.