Bridging the Gap Between Policy and Practice: The Rise of Enforceable Data Governance
10 minutes
May 23, 2025
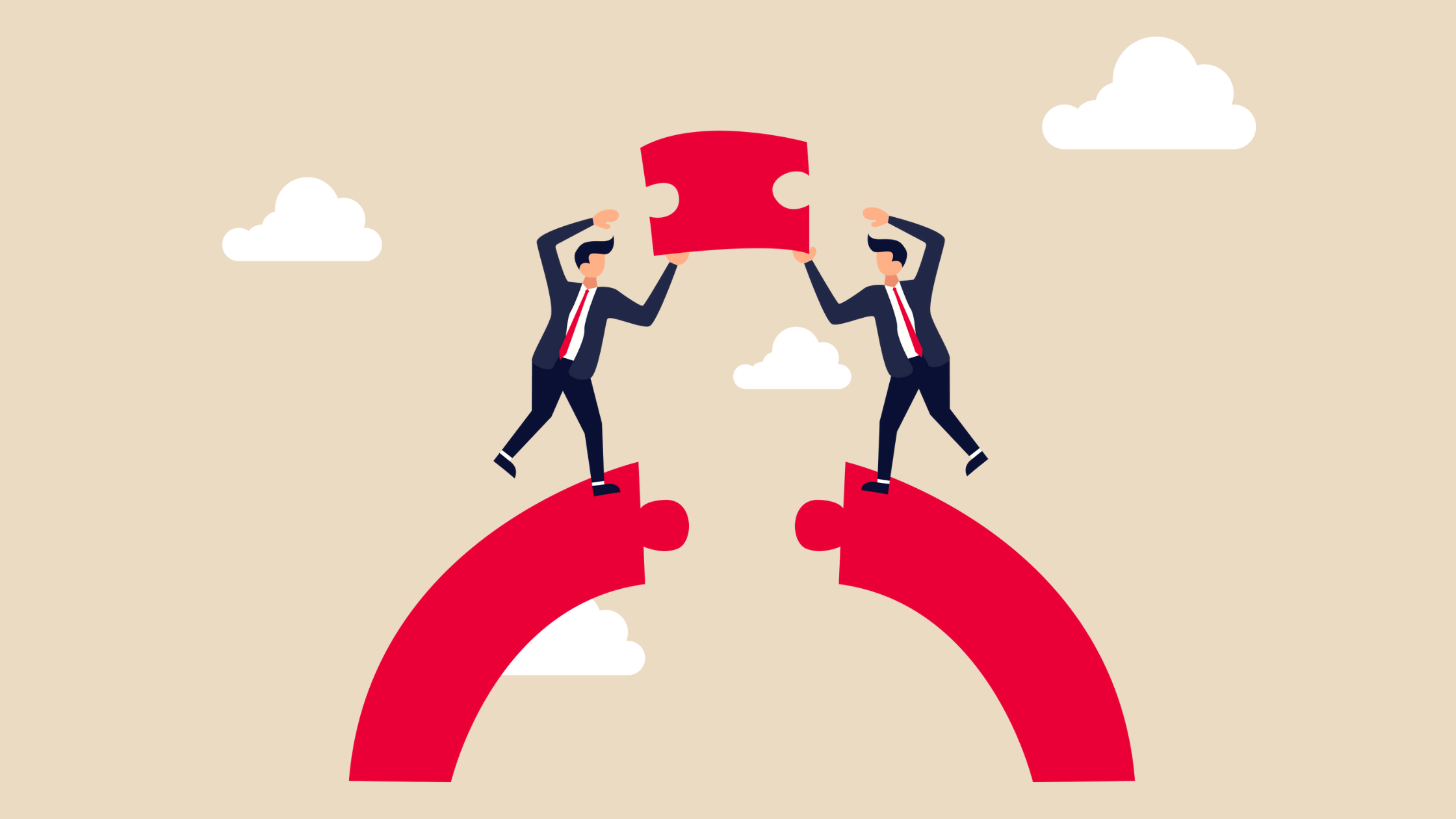
In today’s digital-first economy, data is not just an asset—it’s the lifeblood of innovation, data driven decision-making, and strategic advantage. As organizations increasingly rely on data to power everything from artificial intelligence models to personalized customer experiences, the effective and responsible data governance has never been more critical.
Yet, data governance often remains trapped in a legacy mindset—seen as a bureaucratic checkbox or a collection of policy PDFs stored in static repositories. This disconnect in data governance, between documentation and operational reality is placing organizations and enterprises at risk of compliance failures, reputational damage, and missed opportunities for responsible innovation.
So how can organizations and enterprises evolve from reactive data governance to a proactive, embedded approach that supports both data compliance and agility?
Let’s explore the paradigm shift towards policy-centric enforcement—a new frontier in data governance that enables real-time control, transparency, and trust.
What is Data Governance?
Data governance is a critical data management strategy designed to enhance data quality, data security, and data accessibility across an organization. It provides a structured framework of policies, standards, and best practices that guide how enterprise data is collected, classified, stored, managed, and utilized.
By establishing clear rules for data ownership, data stewardship, and compliance, data governance ensures the accuracy, integrity, and privacy of sensitive information, especially in regulated industries.
Strong data governance practices are essential for supporting data-driven decision-making, maintaining regulatory compliance (like GDPR, HIPAA, and CCPA), and enabling secure cloud data environments and AI-ready infrastructure.
Why Traditional Data Governance Fails in the Age of AI, Cloud, and Real-Time Analytics
For decades, enterprises have approached data governance with frameworks built for an era of static systems and tightly controlled environments. Legacy governance models focused on:
- Data Cataloging: Building and maintaining inventories of enterprise data assets
- Policy Documentation: Crafting rules, guidelines, and compliance procedures for data use
- Manual Data Access Controls: Managing permissions through centralized IT or governance councils
- Scheduled Compliance Audits: Retrospective reviews to ensure regulatory and internal compliance
These pillars were effective when enterprise data resided in centralized databases, accessed by limited users, and when technology evolved at a slower pace.
But the data landscape has changed dramatically.
The Shift to Modern Data Ecosystems
Today’s organizations are powered by distributed data architectures, real-time pipelines, and AI-driven insights. Data is no longer confined to a single warehouse or platform, it flows across hybrid cloud, multi-cloud environments, and decentralized business units.
Key trends disrupting traditional data governance include:
- Massive data volume and variety across structured, semi-structured, and unstructured sources
- AI and machine learning workflows that pull and process sensitive data continuously
- Self-service analytics platforms enabling non-technical teams to explore datasets independently
- Domain-oriented data ownership, where business units manage their own data governance policies
This shift has rendered many legacy governance practices inadequate. The traditional model designed to enforce control struggles to adapt to the speed, scale, and flexibility required in modern data-driven organizations.
The Need for Agile, Scalable Data Governance
Successful organizations are moving toward adaptive data governance models that are:
- Automated: Real-time policy enforcement, dynamic access control, and continuous monitoring
- Decentralized: Enabling domain-specific governance aligned to business contexts
- AI-aware: Incorporating governance into AI/ML pipelines from data sourcing to model output
- Cloud-native: Designed to operate seamlessly across cloud and hybrid infrastructures
To remain competitive and compliant, businesses must rethink how they manage data privacy, access, security, and quality not as a checkbox, but as an embedded layer across every data process.
Why Legacy Approaches to Data Management No Longer Work?
1. Static Policies with No Enforcement
Most data governance programs still revolve around documentation—PDFs, SharePoint wikis, or spreadsheets that define who can access what and under which conditions. But these policies often sit idle, detached from actual data systems. Without a mechanism to actively enforce them at the point of access or usage, they rely on human discipline—an unreliable and risky approach in fast-paced environments.
2. Lack of Operational Control
Governance teams frequently lack visibility into how data is being accessed or used after access is granted. Who accessed which dataset? Was it for an approved purpose? Was any sensitive data downloaded or exported? Traditional systems often can’t answer these questions in real time. This lack of observability not only hampers compliance monitoring but also limits the ability to respond to misuse before it escalates.
3. Fragmented Toolchains
Modern data architectures span multiple platforms—cloud data warehouses, SaaS applications, on-prem systems, and third-party APIs. Each of these may have its own access controls, logging mechanisms, and data classification schemes. Without a unified policy enforcement layer, governance teams are forced to cobble together oversight through disconnected tools, increasing the risk of inconsistent enforcement and missed gaps.
4. Delayed Response to Non-Compliance
Even when data governance issues are detected, they’re usually discovered during quarterly audits or through incident reports, often after the damage is done. The reliance on manual, retrospective data auditing makes it nearly impossible to address compliance violations in a timely manner. In high-risk industries, this delay can lead to regulatory penalties, reputational damage, and legal exposure.
Governance Fatigue: When Compliance Slows Down Data Teams
These limitations not only compromise data privacy compliance—they also stifle data-driven innovation.
Teams eager to leverage data for real-time analytics are often stuck waiting on approvals or working around legacy data governance models . Meanwhile, data governance teams—overwhelmed by big data environments —lack the bandwidth and tooling to scale.
This creates a dangerous paradox: governance is either seen as a bureaucratic blocker or completely bypassed, turning into a creating security and compliance risks . Neither scenario is sustainable in a data-driven organization.
The solution lies in reimagining governance not as static oversight, but as embedded enforcement—proactively enabling data usage while ensuring every action is policy-aligned and auditable.
Why Policy-Centric Data Governance Is Key to Scalable Automation
In legacy data governance models, organizations often rely on manually documented policies and manual compliance checks . While these methods have worked in on-premise data systems ], they fall short in real-time analytics pipelines.
Enter automated policy enforcement in data governance —a model that operationalizes governance at scale across hybrid data infrastructures by embedding enforcement directly into data workflows and AI/ML pipelines
Instead of relying on users to follow rules, this model makes the system responsible for applying governance policies automatically such as data access control, lineage tracking, and PII masking, consistently, and in real time across cloud-native and self-service environments.
What Policy-Centric Enforcement Looks Like in Practice
Let’s break this down with real-world examples:
1. Automated Access Controls Based on Role, Purpose, or Sensitivity
Example:
A data scientist at a healthcare company needs access to patient records for a predictive modeling project.
- Without policy-centric enforcement: They submit a ticket, wait for approval, and might gain full access to raw data—even more than needed.
- With policy-centric enforcement: The system checks the user’s role, confirms the approved project purpose, and grants access only to the necessary fields (e.g., de-identified patient data), automatically denying access to sensitive PII like names or addresses.
2. Real-Time Data Masking or Redaction
Example:
A business analyst from the marketing team runs a query on the customer database.
- Policy-centric enforcement detects:
- The analyst isn’t part of the finance or compliance teams
- The request comes from a non-secure device
- Outcome: Fields like credit card numbers and SSNs are automatically masked, while non-sensitive attributes like age range and purchase history remain visible.
3. Auditability for Every Data Access Request
Example:
A third-party contractor working on churn prediction accesses user data from a cloud data warehouse.
- The system logs:
- Who made the request
- What data was accessed
- When, where, and why it was accessed
- Whether masking/redaction was applied
- If a regulator later asks for proof of compliance with GDPR, the organization can provide a full, timestamped audit trail—automatically.
4. Dynamic, Context-Aware Governance Decisions
Example:
An employee traveling abroad attempts to access internal HR data using public Wi-Fi.
- Policy-centric enforcement:
- Recognizes the device is not compliant
- Sees access is happening outside standard work hours
- Detects that the IP is coming from a high-risk region
- Result: The system dynamically blocks access and alerts the governance team—without requiring human intervention.
Key Benefits of Automated Policy Enforcement in Modern Data Governance
As data grows in volume, velocity, and variety, l legacy data governance methods are no longer enough.Data governance teams and professionals can no longer rely on manual compliance documentation and data audits alone—they need automated data governance tools that are dynamic, intelligent, and embedded across the data lifecycle.
Modern platforms like OneTrust’s Data Policy Enforcement are designed to meet this challenge head-on. By embedding machine-readable data governance policies directly into the enterprise data fabric, they empower governance professionals to shift from passive oversight to proactive, automated control.
Here’s how policy-centric enforcement drives real, tangible benefits across the organization:
1. Operationalizes Data Governance
Too often, governance policies live in static documents or compliance portals—disconnected from the systems that handle data.
Policy enforcement turns governance from theory into practice.
- Example: A policy stating “PII data should not be accessed by contractors” isn’t just written—it’s enforced automatically at the moment a contractor tries to query a PII-containing table.
- Outcome: Governance teams gain confidence that rules are being applied consistently, without requiring constant manual intervention.
2. Accelerating Innovation While Ensuring Data Security
One of the biggest tensions in modern data-driven companies is between speed and security. Data users want agility, while governance wants control.
Policy enforcement bridges this gap by allowing governed self-service.
- Example: A data scientist needs customer data to train a churn model. Instead of waiting days for approvals, they get instant access to a governed version of the dataset—with sensitive fields masked and usage logged.
- Outcome: Innovation moves faster, while governance ensures compliance and privacy controls are upheld.
3. Enhances Risk Management with Greater Visibility and Control
Without real-time enforcement, most organizations are blind to how data is used across cloud systems, analytics platforms, and user endpoints.
Policy-centric enforcement creates a transparent, auditable data environment.
- Example: Every data access event is logged—who accessed it, when, from where, for what purpose—and compared against defined policies.
- Outcome: Governance teams can proactively detect policy violations, respond to anomalies, and demonstrate due diligence to regulators.
4. Streamlining Regulatory Compliance for Data-Driven Organizations
With regulations like GDPR, CCPA, HIPAA, and more evolving constantly, compliance is a moving target.
Policy enforcement aligns operational practices with regulatory mandates—by design.
- Example: A policy blocks the export of EU resident data to non-compliant regions. If someone tries to run such a query, the platform automatically denies the request or applies anonymization.
- Outcome: Organizations ensure continuous compliance without needing manual data reviews or legal escalations for every use case.
5. Enables Cross-Team Collaboration on a Common Framework
Data governance is not the job of one team. It spans legal, privacy, security, data engineering, and business units.
Policy-centric enforcement provides a shared language and system of control.
- Example: Privacy teams define data handling policies, engineers implement them via APIs, and analysts interact with governed datasets—all through a centralized policy engine.
- Outcome: Silos are broken. Everyone works from a single source of truth, increasing accountability, reducing friction, and improving data culture across the board.
Real-World Use Case: From Data Discovery to Purpose-Based Access
Let’s say an analyst wants to access customer data for a retention campaign. With policy-centric enforcement in place:
- The platform checks the business purpose ("retention analysis") against predefined data access rules.
- If the analyst’s role is authorized and the data sensitivity aligns, access is automatically granted—perhaps with masking of PII.
- The access event is logged, auditable, and expires after the campaign ends.
No emails. No Excel trackers. No manual approvals. Just clean, compliant access, every time.
Looking Ahead: Building Trust Through Enforceable Governance
As data grows in complexity and value, so does the need to govern it responsibly. Policy-centric enforcement is not just a technical evolution—it’s a cultural shift towards building trust into every data decision.
Organizations that embed enforceable governance into their data operations will be better positioned to:
- Foster ethical AI development
- Respond to regulatory changes with agility
- Build customer trust and loyalty
- Drive innovation without compromising privacy
Final Thoughts
Policy-centric enforcement is the future of scalable, responsible data governance. It empowers governance teams with the tools they need to not just define policies, but to actually enforce them—automatically, intelligently, and in real time.
As platforms like OneTrust continue to pioneer this space, organizations must rethink how they embed governance into the very DNA of their data ecosystems. Because in a world where data is power, enforceable governance is the key to using it wisely.
SHARE THIS
Discover More Articles
Explore a curated collection of in-depth articles covering the latest advancements, insights, and trends in AI, MLOps, governance, and more. Stay informed with expert analyses, thought leadership, and actionable knowledge to drive innovation in your field.
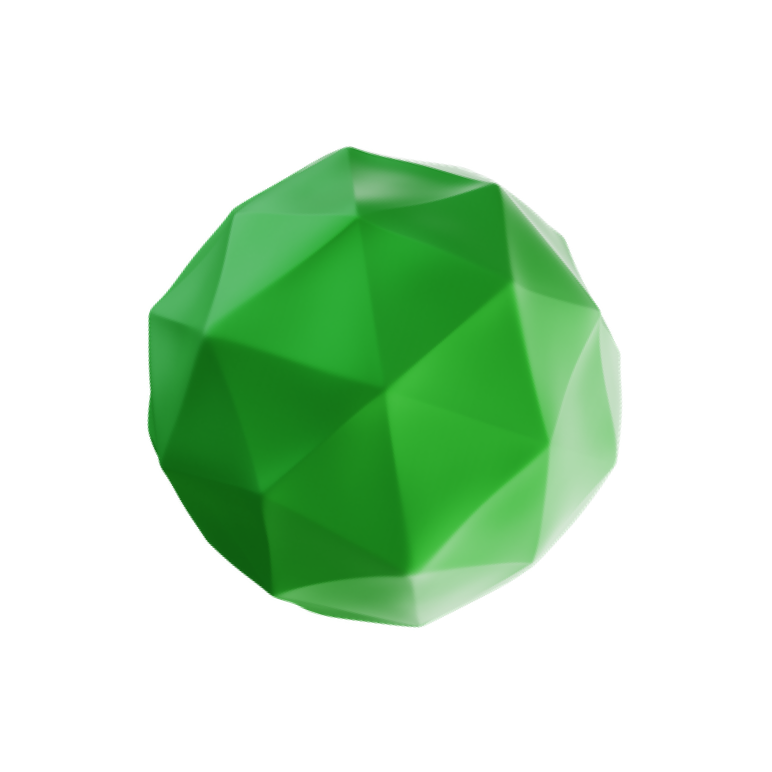
Is Explainability critical for your AI solutions?
Schedule a demo with our team to understand how AryaXAI can make your mission-critical 'AI' acceptable and aligned with all your stakeholders.