AI Privacy in the Age of Acceleration
10 minutes
May 19, 2025

In an era where artificial intelligence is transforming how we work, live, and govern, one principle remains non-negotiable: privacy concerns with AI. As data becomes the currency of innovation, the risks surrounding its misuse have never been higher. From healthcare records to behavioral patterns, from biometric identifiers to personal messages, AI systems today are built on data that is deeply personal. With the rapid deployment of machine learning models across industries, concerns about data privacy, misuse, and ethical governance are no longer theoretical; they are urgent, real-world challenges.
But why is AI Privacy a concern?
AI privacy shares deep roots with the broader concept of data privacy. Data privacy, also referred to as information privacy, centers on the idea that individuals should maintain authority over their own personal information. This includes having the right to determine how their data is gathered, stored, and used by organizations. However, while data privacy has been a long-standing concern, the rise of artificial intelligence has significantly reshaped how it's perceived and applied, introducing new complexities and considerations.
Hence the balance between AI innovation and AI privacy has become increasingly difficult to strike. The AI privacy threat landscape is novel, complex, and not effectively addressed by traditional solutions. Privacy teams, often stretched thin and operating with limited resources, face an uphill battle. As AI regulatory and governance pressure mounts, businesses are compelled to prioritize privacy, but many still struggle with the slow pace of compliance workflows, heavy manual processes, and the complex task of keeping up with rapid technological change.
This blog explores the evolving landscape of AI privacy—a critical consideration as businesses and governments embrace intelligent systems to transform operations, decision-making, and engagement, while managing the myriad privacy risks inherent in these technologies.
How does AI track you?
Over the last decade, perceptions of data privacy have undergone a dramatic shift. What once seemed like a minor tradeoff—sharing online shopping behavior for personalized ads—has evolved into a broader societal concern about how AI models learn from, replicate, and expose personal data. AI privacy concerns are not just about protecting data but understanding how AI systems interact with it. Unlike traditional data systems, new age artificial intelligence models don’t just process data—they learn from it, memorize patterns, and potentially reproduce private or protected content in unexpected contexts. With more sensitive data being collected, stored and processed to train generative AI models, the odds of data leakage or infringement, in a way that it violates privacy rights are greater than ever. As Jennifer King from Stanford University puts it, “We’ve seen companies shift to ubiquitous data collection that trains AI systems, which can have major impacts across society, especially on our civil rights.”
The systems we build now won’t just reflect today’s norms—they will shape the digital boundaries of tomorrow.
What Is AI Privacy and Why Does It Matter Now?
AI privacy refers to the protection of personal or sensitive information used by AI systems. While rooted in traditional data privacy, the scale, autonomy, and opacity of AI make its risks far more complex. A decade ago, privacy concerns revolved around ad tracking and e-commerce data. Today, AI systems collect and learn from vast datasets—emails, medical records, voice, and biometric data—often without explicit consent. This data is then used to train models that make decisions about hiring, lending, policing, and more. AI no longer just personalizes shopping recommendations—it can determine life opportunities. With little transparency or accountability, people often don’t know how decisions are made, or whether their data played a role. AI privacy matters now because the stakes are no longer commercial—they’re societal. It's about ensuring autonomy, dignity, and civil rights in a world where machines learn everything about us.
Understand Key AI Privacy Risks
AI privacy teams today are grappling with various emerging risks as AI systems become more pervasive. Our research has identified six key categories of AI related privacy risk:
- Collection of Sensitive Data – AI models require vast datasets, which inevitably include sensitive information. This could include health records, biometric data, and financial logs that, if mishandled, can lead to breaches.
- Consentless Data Gathering – AI systems are often trained on data scraped from the web without explicit consent. This includes everything from resumes to photos that were shared for a different purpose.
- Purpose Creep & Unauthorized Use – Data shared for one purpose may be used for another without user approval, violating trust.
- Unchecked Surveillance and Bias – AI can amplify surveillance systems, leading to biased decisions, especially in law enforcement contexts.
- Data Exfiltration – Hackers target AI systems to gain access to private documents or confidential data, introducing new risks for organizations.
- Data Leakage from AI Outputs – Even well-meaning AI models can accidentally expose private data, creating unintended privacy violations.
The AI Privacy Dilemma: Innovation vs. Compliance
The digital economy runs on data. It’s the invisible fuel behind personalized shopping experiences, real-time fraud detection, predictive healthcare, and the ever-evolving capabilities of AI. For modern businesses, leveraging data isn’t optional—it’s essential for staying competitive and delivering value.
But as AI systems grow more powerful and data-hungry, so too does the ethical and legal responsibility to protect that data. Consumers and regulators alike are demanding higher standards of transparency, accountability, and consent. Privacy is no longer a side concern—it’s a core pillar of trust in the age of AI.
Regulatory frameworks such as the General Data Protection Regulation (GDPR) in Europe, the California Consumer Privacy Act (CCPA) in the U.S., and the upcoming AI Act in the EU, have all significantly raised the bar for privacy compliance. These laws place strict conditions on how data is collected, processed, shared, and stored—especially when it comes to AI systems that learn from and act on personal information.
However, despite growing AI regulation, privacy and compliance teams often remain under-resourced. In many organizations, they still rely on manual processes—reviewing data maps, ticking off compliance checklists, and conducting long-winded risk assessments. These teams are charged with enforcing guardrails but often lack the tools, automation, or influence to keep pace with innovation.
Meanwhile, product, data science, and engineering teams operate on a different timeline—one driven by rapid iteration, competitive pressure, and the mandate to innovate. Their goal is to deploy new AI features and capabilities fast, often viewing privacy as a blocker rather than a strategic enabler.
This misalignment between compliance culture and innovation culture creates friction:
- Privacy reviews delay product launches.
- Engineers may bypass risk assessments to meet deadlines.
- Sensitive data might get exposed, used without consent, or fed into AI systems without proper governance.
The result? Privacy failures that damage user trust, attract regulatory scrutiny, and harm brand reputation.
Worse yet, when breaches or misuse occur, companies risk more than just fines—they risk eroding their license to operate in an AI-driven economy.
The real challenge lies in bridging this gap—making privacy not just a checkbox or a barrier, but a built-in feature of AI development. Organizations that succeed will be those that treat privacy as a design principle, embedding it into the AI lifecycle from data sourcing and model training to deployment and monitoring.
Ultimately, the privacy dilemma isn’t about choosing between innovation and compliance. It’s about integrating both. In a world where trust is currency, responsible AI isn’t just good ethics—it’s good business.
AI for Privacy, Not Just Privacy for AI
Artificial Intelligence—when built responsibly—can be a force multiplier for privacy programs. AI can:
- Automate Repetitive Compliance Tasks: From data inventory mapping to privacy impact assessments (PIAs), AI can dramatically reduce the manual overhead on privacy teams.
- Enhance Risk Detection: ML algorithms can monitor data usage across systems in real-time and flag potential compliance breaches before they escalate.
- Enable Dynamic Governance: Privacy isn’t static. New regulations, new data types, and new applications evolve constantly. AI can continuously learn and adapt governance policies without starting from scratch every time.
Moving From Manual Compliance to Intelligent AI Privacy Ops
Traditionally, privacy workflows involve checklists, documentation, and slow coordination between legal, IT, and product teams. AryaXAI is helping organizations leapfrog these limitations by introducing intelligent privacy operations. Here’s how:
1. AI-Powered Data Discovery & Classification
Understanding what data you have, where it resides, and how it flows is foundational to privacy. Our tools leverage NLP and computer vision to scan structured and unstructured data sources—identifying PII, PHI, and other sensitive fields. This reduces blind spots and lays the groundwork for responsible AI deployments.
2. Context-Aware Risk Assessment Engines
Not all data processing is equally risky. AryaXAI's intelligent assessment engine weighs the context—purpose, sensitivity, regulatory environment—to prioritize privacy risks effectively. This allows privacy teams to focus efforts where it matters most.
3. Autonomous Compliance Agents
Imagine having a virtual privacy analyst embedded in every product sprint or deployment cycle. AryaXAI enables this through autonomous AI agents that monitor product pipelines, review data usage, and enforce privacy policies automatically—scaling governance without scaling headcount.
4. Real-Time Policy Adaptation
When regulations change, your governance should too. AryaXAI leverages large language models (LLMs) to parse new regulations and align internal policies. This means your systems stay compliant in real-time, not just during annual audits.
The unique privacy challenges of AI
As AI technology advances, privacy teams are under increasing pressure to ensure compliance and mitigate risks while managing the complexities of modern data processing systems. These teams are tasked with navigating an evolving regulatory landscape, managing vast amounts of sensitive data, and ensuring the ethical use of AI—all while balancing the need for innovation. Here are some key challenges that privacy teams are currently facing:
1. Complex Regulatory Compliance
The regulatory environment surrounding data privacy is rapidly evolving, with frameworks like the GDPR and the upcoming AI Act imposing stricter requirements. Navigating these complex regulations requires privacy teams to constantly stay updated on new rules, ensuring their company remains compliant. For example, AI's capability to use personal data in unexpected ways adds layers of complexity to regulatory compliance, especially when dealing with cross-border data transfers.
2. Lack of Resources and Expertise
Privacy teams are often underfunded and understaffed, especially in smaller organizations. The rapid pace of technological change means that privacy professionals must continuously learn new tools, technologies, and regulatory requirements, a task that becomes even more difficult when resources are limited. Many teams struggle to keep up with AI advancements that directly affect privacy, such as data scraping, machine learning algorithms, and automated decision-making systems.
3. Increased Volume and Variety of Data
AI models rely on vast amounts of diverse data, much of which can be personal or sensitive. Privacy teams are tasked with managing this data, ensuring it is properly anonymized, secured, and used in compliance with privacy regulations. However, the sheer scale and variety of data sources—from social media to medical records—make it difficult to track where and how data is used, and ensure all regulations are being followed.
4. Opacity of AI Models
One of the biggest concerns for privacy teams is the lack of transparency in many AI models. Often referred to as “black box” systems, these models learn from data in ways that are not always explainable, making it challenging to assess how personal data is being used or whether it is being exploited. This opacity not only makes it difficult to ensure compliance but also raises concerns about the potential for biased or discriminatory outcomes.
5. Ensuring Data Minimization in AI
Data minimization is a core privacy principle that suggests only collecting the minimum amount of personal data necessary for a specific purpose. However, many AI systems are designed to collect and process large amounts of data to improve performance. Privacy teams must find a way to ensure that data collected for AI training purposes is not excessive, remains relevant, and is securely anonymized where possible.
6. Managing Data Breaches and Security Risks
As AI systems often aggregate massive datasets, they also become prime targets for cybercriminals. Privacy teams must be proactive in ensuring that data is secure and that any breaches are swiftly identified and mitigated. The risks of data exfiltration, malicious AI manipulations, or accidental exposure are ever-present, and privacy teams need to have robust protocols in place for breach management.
7. Balancing Innovation with Privacy
As organizations rush to adopt AI to stay competitive, privacy often becomes a secondary concern. Privacy teams face the challenge of ensuring that privacy protection doesn’t stifle innovation. Striking the right balance between the need to collect and use data for AI-driven insights, while also maintaining user privacy, requires constant negotiation between the legal, technical, and business sides of the organization.
8. Stakeholder Misalignment
Privacy teams must work closely with other departments—such as legal, engineering, and marketing—to ensure privacy is embedded in every stage of AI development. However, these teams often have conflicting priorities. For instance, business teams may push for faster product launches, while engineering teams may prioritize AI optimization over privacy considerations. Aligning all stakeholders on privacy goals and ensuring that privacy is not compromised for speed or profits is a critical challenge.
Building AI That Respects Privacy by Design
As AI continues to redefine the way we live, work, and interact with the world, privacy must evolve from an afterthought to an embedded principle. The road ahead requires a paradigm shift—from reactive compliance to proactive, intelligent privacy engineering.
At AryaXAI, we envision a future where privacy is not a bottleneck to innovation, but a catalyst for trustworthy AI. This means operationalizing privacy through intelligent tools, aligning stakeholders across compliance and engineering, and embedding privacy safeguards at every layer of the AI lifecycle.
The challenges are real—rising regulatory complexity, ever-expanding datasets, and increasing public scrutiny. But so are the opportunities. Organizations that invest in AI-native privacy infrastructure today will lead tomorrow’s trusted digital economy.
As we move forward, the mandate is clear:
- Architect AI systems that respect user agency and consent.
- Build transparency into model behavior and data usage.
- Empower privacy teams with automation and intelligence, not just documentation.
- Foster cross-functional collaboration between compliance, product, and engineering.
The next wave of AI will be defined not just by what it can do—but by how responsibly it does it.
SHARE THIS
Discover More Articles
Explore a curated collection of in-depth articles covering the latest advancements, insights, and trends in AI, MLOps, governance, and more. Stay informed with expert analyses, thought leadership, and actionable knowledge to drive innovation in your field.
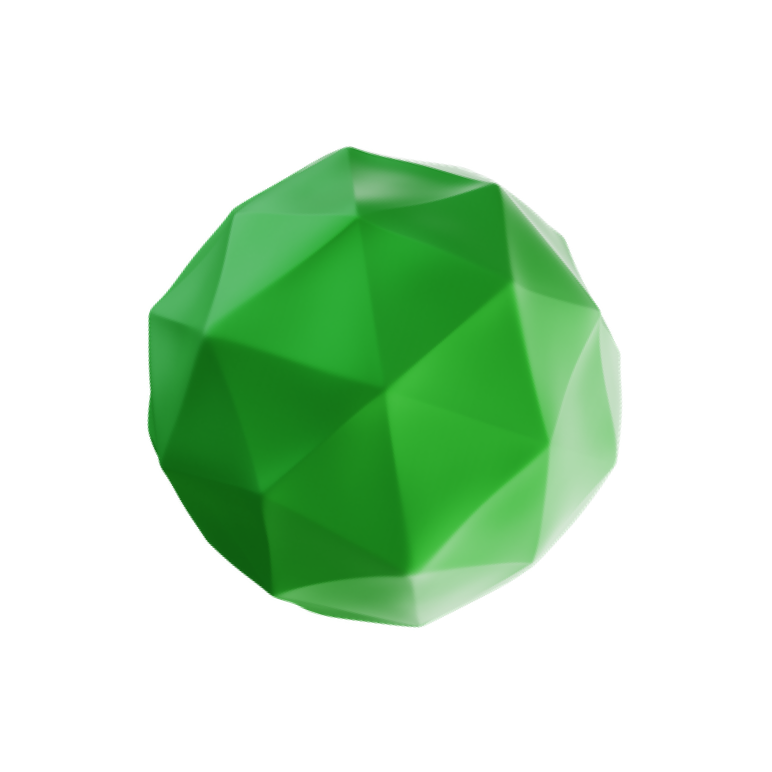
Is Explainability critical for your AI solutions?
Schedule a demo with our team to understand how AryaXAI can make your mission-critical 'AI' acceptable and aligned with all your stakeholders.