Enterprise-grade model auditing


Develop safeguard controls
Perform in-depth audit around ML artecrafts
Understand the value of your ML models, uncover business risks and develop safeguard controls to avoid risks. Integrate fairness, explainability, privacy, and security assessments across workflows.
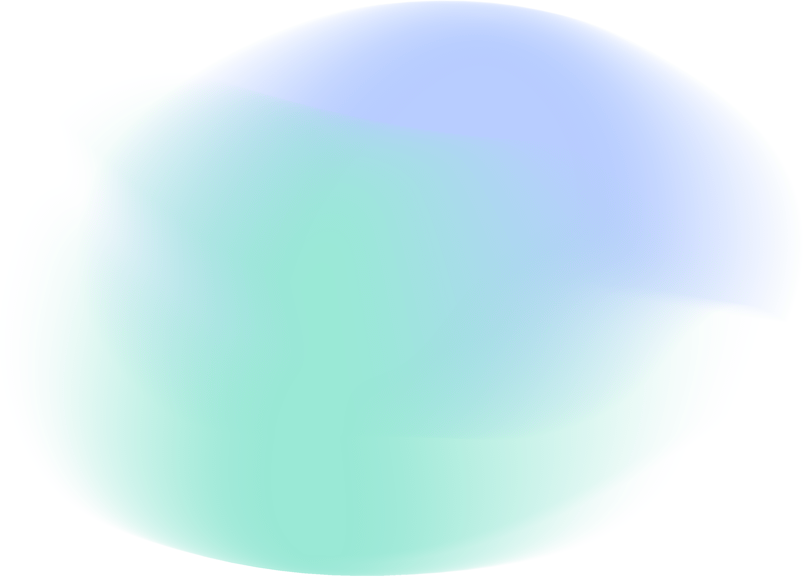
Perform in-depth audit around ML artefacts
For mission-critical use cases, ML auditing ensures systematic execution of processes to identify associated risks and develop safeguard controls to avoid risks. AryaXAI methodically captures various auditable artefacts during training and production.
Organizations can define audit cadences and execute them automatically in AryaXAI. Users can review these reports anytime and anywhere. The key observations can be shared between teams to inform all stakeholders about the gaps and execute the needful corrections. And when it is required to share critical information with regulators or compliance teams, AryaXAI helps aggregate critical information in a jiff.
Data Preparation
Data Selection
- Which data is selected?
- The retionale behind the chice.
- Selection authority
Data Preparation
- Detail about data removal
- Bais mitigation
- Trueness representaition
Feedback Gathering
- Selection of retraining data
- Analysis od errors
- Case-wise true labels
Model Building
Model training
- Technique Selection
Model training
Final model
Model debug
- Validating explainability
- Review of gobal explanations
- Sufficency of explanations
Model Testing
Model Training
Model challenger deifinition
Records of challenger performance
Segmentations/performaces
Test Data
- Sufficiency of test scenarios
- Failure analysis
- Usage risk estimation
- Business risk
Sucess Criteria
- Defining sucess criteria
- Simulated Usage
- Benefits estimation
- Sign off authorization
Model Testing
Case-wise
- Predictions record
Model state
Local explainability
Model consistency
- Event of data drift
- Events of model drift
- Records of biased predictions
- Records of model degradation